Guest post by Taym Alsalti. If you want a citable version, see this preprint with Jamie Cummins & Ruben Arslan.
Okay, maybe not require require, but it would help immensely. As the title very subtly implies, this issue is a special case of the more general Statistical Control Requires Causal Justification. A slightly longer tl;dr than the title is: Responses flagged as careless are routinely removed from data sets before conducting the main analyses (one type of “statistical control”, conditioning the analyses on the absence of “carelessness”). Intuitively, this makes sense (less bad data => better data overall), and sometimes purging careless responses is the right thing to do (when careless responding is a confounder). But other times, ignoring such responses makes more sense as excluding them would increase rather than decrease bias (e.g., when careless responding is a mediator, collider, or both).
Que’ce que c’est and why you should care about it
“Careless” is usually used to describe specific kinds of naughtiness, such as straightlining responses on a survey page or choosing responses “randomly”. The causal principles we discuss here definitely apply to such a specific understanding of careless responding (CR), but not only. We thus use CR to refer to any pattern of responding that reflects a latent construct which is distinct from, and interferes with the measurement of, the targeted construct [1]Many many other terms are used in the literature, e.g., inattentive, insufficient effort, lazy, random, disengaged, nonserious, mischievous, bogus, untruthful, fraudulent, etc. The question of how much these meaningfully overlap is interesting, but not the focus here.. CR is caused by person (e.g., personality), study (e.g., boringness), and context (e.g., what music the participant is listening to while taking the study). So this definition encompasses a variety of non-random response patterns , such as acquiescence bias, central tendency bias, socially desirable responding, and intentionally fraudulent responses.
Measures to ensure data fidelity predate the public world wide web (e.g., the MMPI validity scales) and are often needed in any human data collection endeavor. However, nowadays people most often complain about CR in the context of anonymous online surveys, where a few major blunders recently generated some buzz, e.g., that one time YouGov/The Economist overestimated the proportion of young people yay-saying the statement “The Holocaust is a myth” by ~17 percentage points (20% by YouGov vs. 3% by Pew). Concerns about data quality issues in online surveys are probably justified, and things seem to be getting worse for surveys that include open-ended questions which are economical to answer using LLM responses. But it’s not time to give up on online studies yet!
How to deal with careless responding?
Well, first we have to measure it. There are many different ways to do that and we refer the reader to Goldammer et al., 2020 and Ward & Meade, 2023 for reviews and guidelines, but standard practice involves using such CR identification measures to form binary decision rules based on which the offenders are list-wise excluded. And it is with this meet-’n-yeet approach as a standard way of dealing with CR that we take issue [2]The dichotomisation of a continuous phenomenon is a problem in and of itself, but we’re picking our fights here. Although we do use the more general “control for” to include alternatives such as weighting using metric measures of CR (see Ulitzsch et al., 2024, for an example) or including them as covariates in a regression analysis. — with few exceptions (e.g., Alvarez & Li, 2023 caution that excluding careless respondents can compromise sample representativeness), the prevailing guideline, at least in online surveys, is that once identified, careless responses should be purged.
The common reasoning behind this approach is that such responses can bias “descriptive” statistics (e.g., sample means) and effect size estimates / measures of associations (e.g., correlations and standardized mean difference) as well as increase measurement error. CR can definitely do these things if we don’t account for it. But here’s the thing: it can also bias our sample estimates if we do account for it. In the following, we show under which conditions this can happen using directed acyclic graphs (DAGs)[3]Well, not 100% kosher DAGs, to be upfront, since they incorporate bidirectional associations (dashed lines), but you can think of these non-kosher elements as annotations of the DAGs proper.. We illustrate and provide some examples for a few basic, simple X → Y constellations (see e.g., Rohrer, 2018, but the same principles apply in the case of more complex causal structures or when no causal effects are targeted at all (e.g., when estimating the population distributions of certain variables or the associations between others).
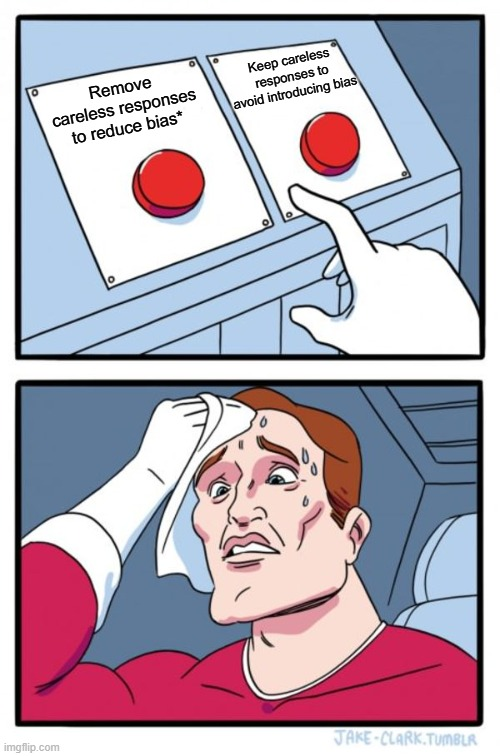
*and measurement error
CR as a confounder
One constellation where controlling for CR is unambiguously necessary is when it causes both our predictor (X) and outcome (Y). The result is a “spurious” or a “spuriously inflated” association between X and Y. In DAG, this pattern is traditionally depicted as a “fork”:
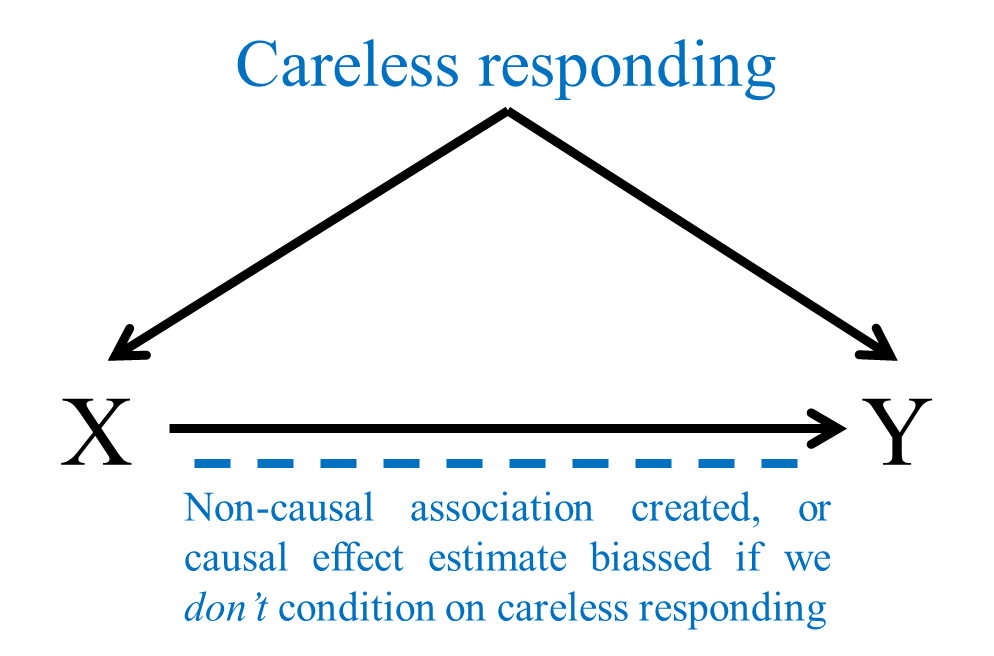
Figure 1: CR as a confounder
One way we can get thusly forked is when the distributions of serious X and Y responses are skewed (such as when they show floor or ceiling effects), while careless responses randomly or systematically cluster around the two scales’ middle points[4]If you’re a visual learner, picture in your mind’s eye a scatter-plot where the vast majority of the points belonging to diligent responders cluster in a small square in the corner of the plot’s canvas, while careless responses cluster in the middle of the plot. This “elongates” the data point cloud and makes the regression line going through it less horizontal. See Stosic et al. (2024) if you want to picture it in your actual eye.. An example is if our X is self-reported frequency of watching InfoWars and Y is the self-reported frequency of bleach ingested to prevent and treat COVID-19. The means (and variances) of serious responses on both X and Y will be very close to the scales’ lower ends, whereas careless responses will either bunch up around the scales’ midpoints (if they’re random) or cluster at the scales’ higher ends (if they’re produced by trolling[5]Little known fact: the English verb “controlling” comes from the Latin “con-troll-are”.).
Random-like or central tendency biased responses can also deflate a true association[6]The cloud in the scatter plot thickens.; extreme responses on either ends of the scales for the sake of trolling can inflate a true association; acquiescence-biased responses can inflate or deflate an association in any direction. The possibilities are not endless but numerous; and all such cases call for conditioning on CR, by for example removing careless responses.
CR as a mediator/collider
Say we have reason to believe that X will influence CR instead of the other way around, i.e., its effect on Y might be partially mediated by CR. In such a case, excluding CR may amount to conditioning on a collider (i.e., a variable that modifies the association between two variables that cause it):

Figure 2: CR as a mediator/collider
This could occur if, for instance, our manipulation (X) is a simple, boring flanker task in the control condition while participants additionally receive musical feedback on their performance in the experimental condition. Here the enrichment of the experimental condition reduces CR compared to the control group, which in turn affects Y (e.g., reaction time). Since we can’t directly manipulate either Y or careless responding[7]Incorporating potential causes of CR into our DAGing is a difficult, but probably worthwhile enterprise. While personality and context can never be completely accounted for, we can attempt to partially get at their impact by, for instance, including a measure of personality which we assume is relevant (e.g., conscientiousness) and explicitly asking about the situation in which the participant is taking the survey. On the other hand, we invariably condition on survey characteristics whether we wish to or not, although there too we can experimentally manipulate some aspects of the survey design (e.g., reward for participation) to investigate their impact., they are likely influenced by variables we do not necessarily measure or account for (U in the DAG), such as the test takers’ personality or the environment in which they’re taking the survey. This so-called backdoor path remains closed (i.e., does not impact our estimate of the effect of X on Y) as long as we restrict our analysis to the bivariate effect of X on Y. But conditioning on CR, e.g., by excluding those deemed as showing it, opens the backdoor path and lets the confounding between CR and Y seep into our estimate of the effect of X on Y.
In the context of experiments this type of collider bias is known as post-treatment bias because it involves conditioning on a variable that is at least partially a descendant of (i.e., affected by) the treatment. For example, say we want to test the effect of ritalin on ADHD symptoms in a new randomized experience sampling method study. It’s likely that more participants in the control group will be flagged for CR than in the experimental condition. Purging CR would thus lead to excluding more of those with the worse symptoms in the control group, leading to an overestimation of the control group symptomatology mean, and consequently a deflated treatment effect estimate[8]Importantly, conditioning on CR is only problematic for internal validity (i.e., validity of the causal inference about the treatment) if we do it based on data collected after the start of the treatment (hence the post-treatment). If we collect data in a pre-trial phase for example, then conditioning on CR is fully harmless – for internal validity. The extent to which excluding the least attentive respondents from a study on ADHD compromises external validity (i.e., generalisability/transportability) is also something that we also have to reckon with..
CR as a collider
There are also many ways we could end up with an inverted fork instead of a fork, with CR becoming a simple collider between X and Y themselves:
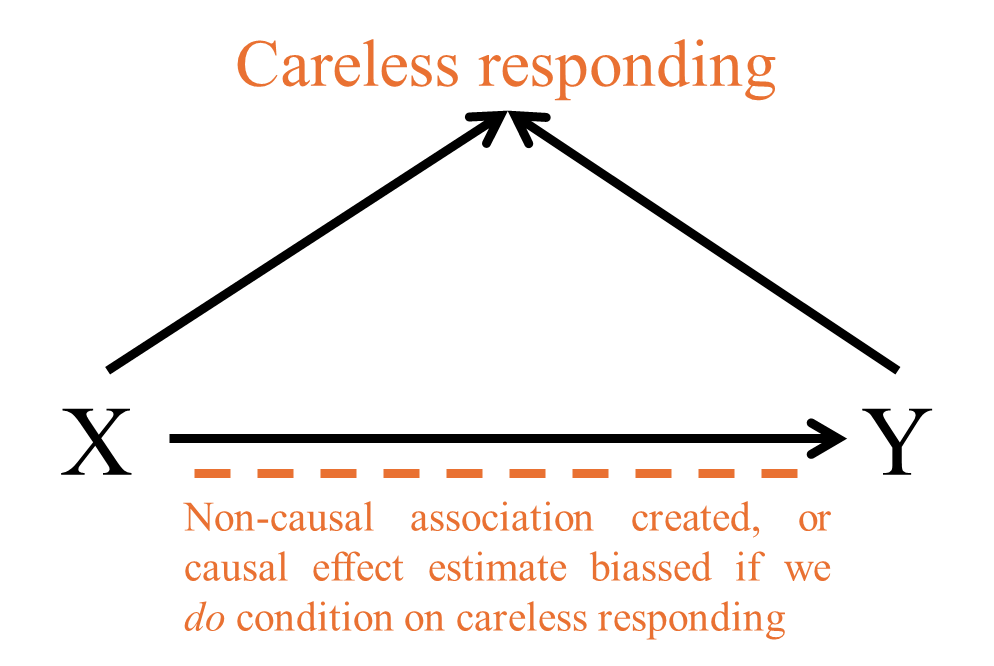
Figure 3: CR as a collider
This could be a problem if we are trying to estimate the effect of, say, conscientiousness (X) on scores of some cognitive test that incorporates an attention component (Y). If we use attention checks to exclude respondents who fail them and reasonably assume that both X and Y affect the probability of such a failure, we might introduce a spurious association between self-efficacy and performance on the cognitive test, or bias the estimate of any true effect that might exist. That is, because diligent responders will tend to be more conscientious and have a stronger latent ability to concentrate, the association of X and Y will be overestimated[9]You’re again invited to picture in your mind’s eye a scatter-plot, now showing a cloud of points strewn all over the place, indicating no association between conscientiousness and cognitive ability. Excluding careless respondents in this case approximately amounts to removing participants in the lower left corner of the plot, which introduces a spurious negative association between the two variables. This is the same example Julia uses when explaining student selection as a form of collider bias..
CR as a descendant/collider
Finally, let’s say X is self-efficacy and Y is scores on a fluid intelligence test. It is reasonable to think of attention span as a facet of fluid intelligence, which would make the likelihood to pass an attention check for example a (partial) descendant of Y (i.e., a variable that is caused by Y)[10]You may have noticed most examples involve attention. Very attentive. This is because CR control is often associated with attention checks but of course the problems we talk about here are not exclusive to attention research but could also occur for other constructs that are related to different types of CR.:
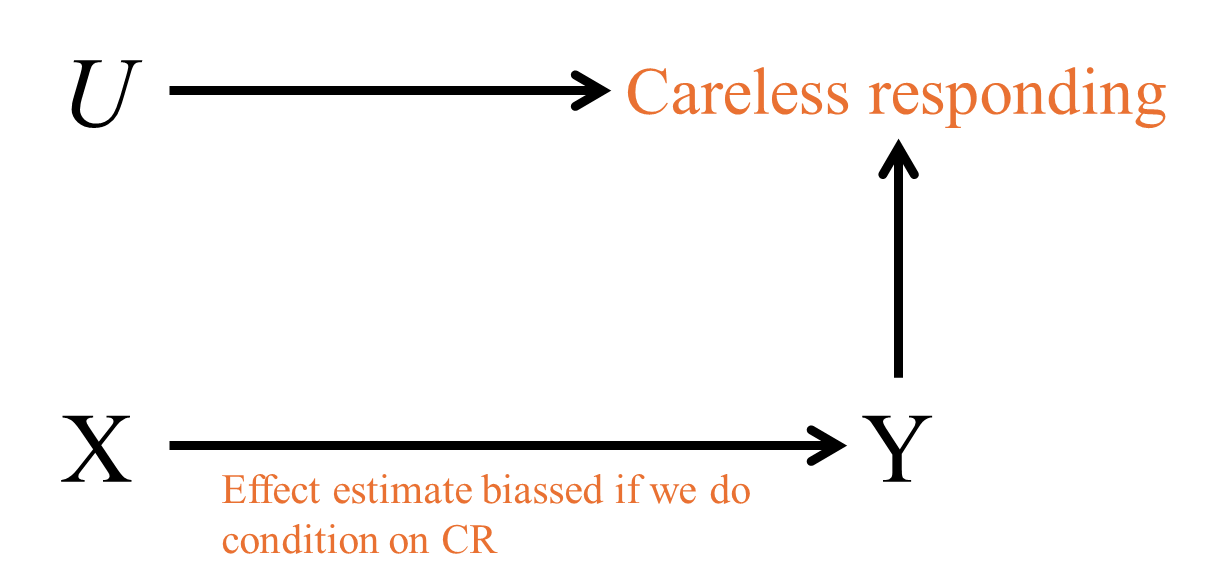
Figure 4: CR as a descendent of the outcome
Conditioning on a descendant of the outcome generally disrupts our effect size estimates in similar ways to conditioning on the outcome itself, i.e., a missing not-at-random (MNAR) scenario. This might not create an association out of thin air like conditioning on a collider (or failing to condition on a confounder) could, but it very well could lead us to miss out on true effects by decimating Y’s sample variance[11]Although this type of range restriction in the outcome only can also inflate effect sizes if we’re talking about “standardized” effect sizes (e.g., Cohen’s d or correlation coefficients, see Alsalti et al., 2024).. In the case of Y as a measure of cognitive ability, this happens because we would be removing those on the lower end of the latent scale.
So what do we actually do about it?
Sadly, there is no easy fix for CR-ridden data. As shown by the examples above, CR can play a role in many different ways, and the best course of action thus always depends on the specific case. Especially when it comes to online studies, how to measure CR and what to do about it are issues that deserve attention and careful thought. A reasonable workflow could look something like this:
- DAG your Xs and Ys
- Consider what types of CR might be plausibly associated with your Xs and Ys and incorporate them into your DAG(s)
- Explore which CR measures are feasible and most likely to reflect the DAGed causal patterns. Do you want to implement external CR detection measures such as instructed response items (e.g., ‘please choose “fully agree”’) and bogus items (e.g., “I am hexagonal shaped”)[12]Pssst they might be redundant at best if you have enough data to calculate ad-hoc measures (e.g., consistency measures, see Yentes, 2020).? Which ad-hoc measures (e.g., long-string index for detecting straightlining) have plausible relationships with your Xs and Ys and what are the relationships’ directions? If you want to exclude careless respondents, decide on thresholds for excluding CR (e.g., failing 3 instructed response items). If you’re fortunate enough to be doing experimental work, make sure you assess CR before the manipulation. That way, it cannot be affected by your X. The remaining concern is that results from your sample of diligent responders might not generalize well to the population, so you’re not scot-free. Also worth noting that applying screening criteria through your data provider (e.g., Prolific or MTurk) also counts as a form of CR exclusion and you have to also consider whether it happens before or after your manipulation, if applicable. If you think controlling for CR might be disadvantageous in your case, do yourself a favor and spell it out in your pre-registration, so readers won’t think it was a post hoc decision. In fact, always spell out how you will deal with CR in your pre-registration.
- Conduct sensitivity analyses. Especially in complex multivariate scenarios (multiple predictors and multiple CR measures), things might turn out to be very different from what you expected (e.g., the prevalence of CR might turn out to be much larger based on the chosen thresholds). In this case, it helps to try out different thresholds for different CR measures and evaluate to what extent conditioning on CR influences the crucial hypothesis tests.
“It’s finally time to think less carelessly about careless responding and acknowledge it requires causal rather than casual thinking” – Julia Rohrer (2025)
Footnotes
↑1 | Many many other terms are used in the literature, e.g., inattentive, insufficient effort, lazy, random, disengaged, nonserious, mischievous, bogus, untruthful, fraudulent, etc. The question of how much these meaningfully overlap is interesting, but not the focus here. |
---|---|
↑2 | The dichotomisation of a continuous phenomenon is a problem in and of itself, but we’re picking our fights here. Although we do use the more general “control for” to include alternatives such as weighting using metric measures of CR (see Ulitzsch et al., 2024, for an example) or including them as covariates in a regression analysis. |
↑3 | Well, not 100% kosher DAGs, to be upfront, since they incorporate bidirectional associations (dashed lines), but you can think of these non-kosher elements as annotations of the DAGs proper. |
↑4 | If you’re a visual learner, picture in your mind’s eye a scatter-plot where the vast majority of the points belonging to diligent responders cluster in a small square in the corner of the plot’s canvas, while careless responses cluster in the middle of the plot. This “elongates” the data point cloud and makes the regression line going through it less horizontal. See Stosic et al. (2024) if you want to picture it in your actual eye. |
↑5 | Little known fact: the English verb “controlling” comes from the Latin “con-troll-are”. |
↑6 | The cloud in the scatter plot thickens. |
↑7 | Incorporating potential causes of CR into our DAGing is a difficult, but probably worthwhile enterprise. While personality and context can never be completely accounted for, we can attempt to partially get at their impact by, for instance, including a measure of personality which we assume is relevant (e.g., conscientiousness) and explicitly asking about the situation in which the participant is taking the survey. On the other hand, we invariably condition on survey characteristics whether we wish to or not, although there too we can experimentally manipulate some aspects of the survey design (e.g., reward for participation) to investigate their impact. |
↑8 | Importantly, conditioning on CR is only problematic for internal validity (i.e., validity of the causal inference about the treatment) if we do it based on data collected after the start of the treatment (hence the post-treatment). If we collect data in a pre-trial phase for example, then conditioning on CR is fully harmless – for internal validity. The extent to which excluding the least attentive respondents from a study on ADHD compromises external validity (i.e., generalisability/transportability) is also something that we also have to reckon with. |
↑9 | You’re again invited to picture in your mind’s eye a scatter-plot, now showing a cloud of points strewn all over the place, indicating no association between conscientiousness and cognitive ability. Excluding careless respondents in this case approximately amounts to removing participants in the lower left corner of the plot, which introduces a spurious negative association between the two variables. This is the same example Julia uses when explaining student selection as a form of collider bias. |
↑10 | You may have noticed most examples involve attention. Very attentive. This is because CR control is often associated with attention checks but of course the problems we talk about here are not exclusive to attention research but could also occur for other constructs that are related to different types of CR. |
↑11 | Although this type of range restriction in the outcome only can also inflate effect sizes if we’re talking about “standardized” effect sizes (e.g., Cohen’s d or correlation coefficients, see Alsalti et al., 2024). |
↑12 | Pssst they might be redundant at best if you have enough data to calculate ad-hoc measures (e.g., consistency measures, see Yentes, 2020). |