Scroll to the very end of this post for an addendum.[1]If you only see footnotes, you have scrolled too far.
Reading skills of children correlate with their shoe size. Number of storks in an area correlates with birth rate. Ice cream sales correlate with deaths by drowning. Maybe they used different examples to teach you, but I’m pretty sure that we’ve all learned about confounding variables during our undergraduate studies. After that, we’ve probably all learned that third variables ruin inference, yadda yadda, and obviously the only way to ever learn anything about cause and effect are proper experiments, with randomization and stuff. End of the story, not much more to learn about causality.[2]YMMV and I hope that there are psych programs out there that teach more about causal inference in non-experimental settings. Throw in some “control variables” and pray to Meehl that some blanket statement [3]“Experimental studies are needed to determine whether…” will make your paper publishable anyway.
Here is the deal though, there is much more to learn about causal inference. If you want to invest more time in this topic, I suggest you take a look at Morgan and Winship’s Counterfactuals and Causal Inference: Methods and Principles for Social Research.[4]Added bonus: After reading this, you will finally know how to decide whether or not a covariate is necessary, unnecessary, or even harmful. If you don’t have the time to digest a whole book[5]I have been informed that only grad students can afford to actually read stuff, which is kind of bad, isn’t it?, read Felix Elwert’s chapter on Graphical Causal Models and maybe also his paper on colliders.
Causal inference from observational data boils down to assumptions you have to make[6]There’s no free lunch in causal inference. Inference from your experiment, for example, depends on the assumption that your randomization worked. And then there’s the whole issue that the effects you find in your experiment might have literally nothing to do with the world that happens outside the lab, so don’t think that experiments are an easy way out of this misery. and third variables you have to take into account. I’m going to talk about a third variable problem today, conditioning on a collider. You might not have heard of this before, but every time you condition on a collider, a baby stork gets hit by an oversized shoe filled with ice cream[7]Just to make sure: I don’t endorse any form of animal cruelty. and the quality of the studies supporting your own political view deteriorates.[8]If you are already aware of colliders, you will probably want to skip the following stupid jokes and smugness and continue with the last two paragraphs in which I make a point about viewpoint bias in reviewers’ decisions.
Let’s assume you were interested in the relationship between conscientiousness and intelligence. You collect a large-ish sample of N = 10,000[9]As we say in German: “Gönn dir!” and find a negative correlation between intelligence and conscientiousness of r = – .372 (see Figure 1).
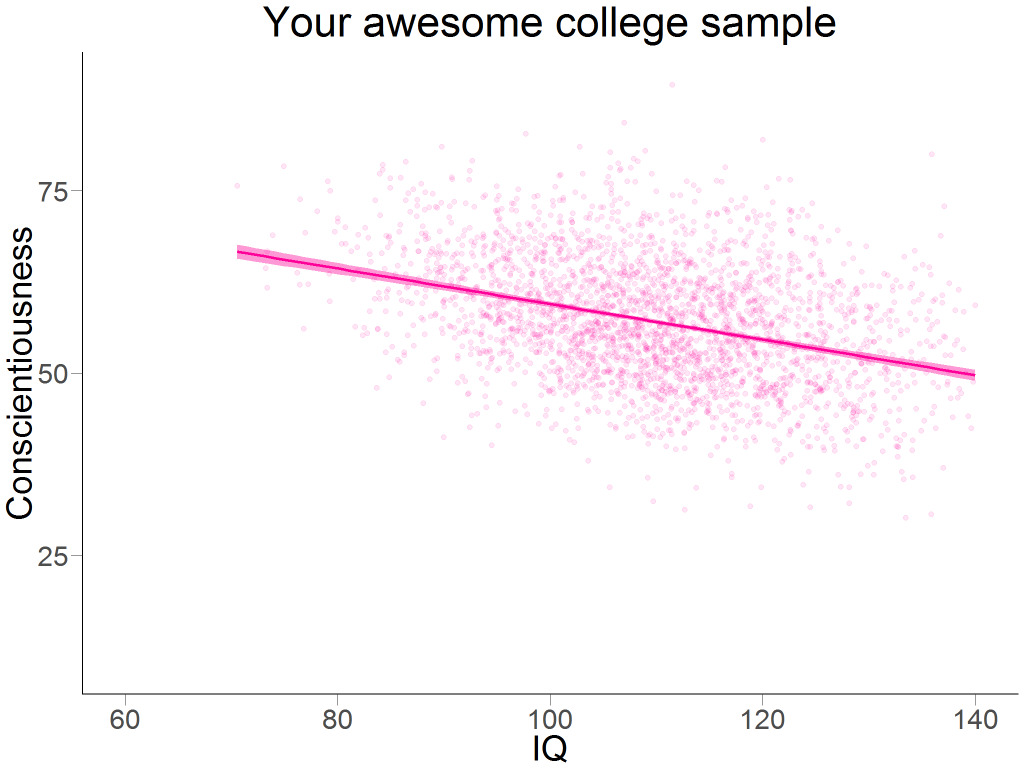
However, your sample consisted only of college students. Now you might be aware that there is a certain range restriction in intelligence of college students (compared to the overall population), so you might even go big and claim that the association you found is probably an underestimation! Brilliant.
The collider – being a college student – rears its ugly head. Being a college student is positively correlated with intelligence (r = .426). It is also positively correlated with conscientiousness (r = .433).[10]Just to make sure: This is fake data. Fake data should not be taken as evidence for the actual relationship between a set of variables (though some of the more crafty and creative psychologists might disagree). Let’s assume that conscientiousness and intelligence have a causal (non-interactive) effect on college attendance, and that they are actually not correlated at all in the general population, see Figure 2.
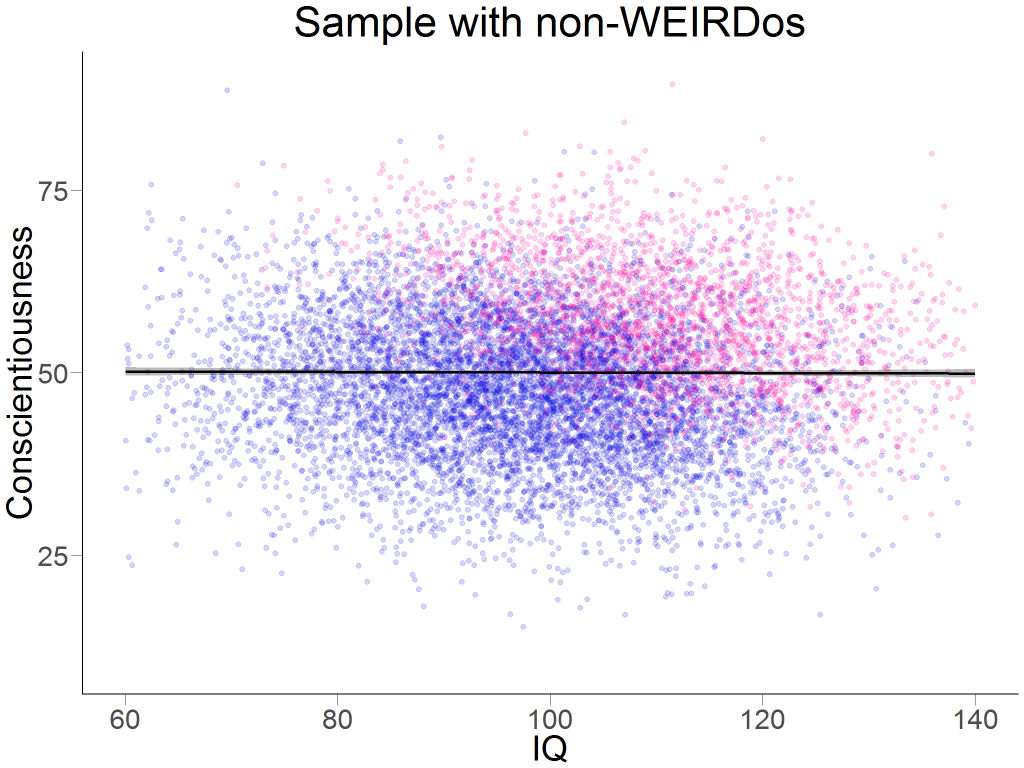
If you select a college sample (i.e. the pink dots), you will find a negative correlation between conscientiousness and intelligence of, guess what, exactly r = -.372, because this is how I generated my data. There is a very intuitive explanation for the case of dichotomous variables:[11]The collider problem is just the same for continuous measures. In the population, there are smart lazy people, stupid diligent people, smart diligent people and stupid lazy people.[12]Coincidentally, you will find each of the four combinations represented among the members of The 100% CI at any given point in time, but we randomly reassign these roles every week. In your hypothetical college sample, you would have smart lazy people, stupid diligent people, smart diligent people but no stupid lazy people because they don’t make it to college.[13]Ha, ha, ha. Thus, in your college sample, you will find a spurious correlation between conscientiousness and intelligence.[14]Notice that you might be very well able to replicate this association in every college sample you can get. In that sense, the negative correlation “holds” in the population of all college students, but it is a result from selection into the sample (and not causal processes between conscientiousness and intelligence, or even good old fashioned confounding variables) and doesn’t tell you anything about the correlation in the general population.
By the way, additionally sampling a non-college sample and finding a similar negative correlation among non-college peeps wouldn’t strengthen your argument: You are still conditioning on a collider. From Figure 2, you can already guess a slight negative relationship in the blue cloud,[15]If you are really good at guessing correlations (it’s a skill you can train!) you might even see that it’s about r = -.200, and pooling all data points and and estimating the relationship between IQ and conscientiousness while controlling for the collider results in r = -.240. Maybe a more relevant example: If you find a certain correlation in a clinical sample, and you find the same correlation in a non-clinical sample, that doesn’t prove it’s real in the not-so-unlikely case that ending up in the clinical sample is a collider caused by the variables you are interested in.
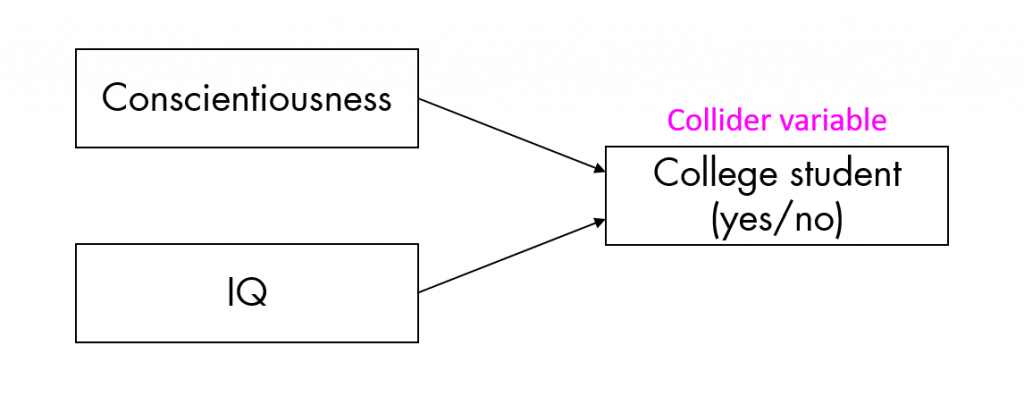
On an abstract level (Figure 3): Whenever X1 (conscientiousness) and X2 (intelligence) both cause Y (college attendance) in some manner, conditioning on Y will bias the relationship between X1 and X2 and potentially introduce a spurious association (or hide an existing link between X1 and X2, or exaggerate an existing link, or reverse the direction of the association…). Conditioning can mean a range of things, including all sort of “control”: Selecting respondents based on their values on Y?[16]or anything that is caused by Y, because the whole collider logic also extends to so-called descendants of a collider That’s conditioning on a collider. Statistically controlling for Y? That’s conditioning on a collider. Generating propensity scores based on Y to match your sample for this variable? That’s conditioning on a collider. Running analyses separately for Y = 0 and Y = 1? That’s conditioning on a collider. Washing your hair in a long, relaxing shower at CERN? You better believe that’s conditioning on a collider. If survival depends on Y, there might be no way for you to not condition on Y unless you raise the dead.
When you start becoming aware of colliders, you might encounter them in the wild, aka everyday life. For example, I have noticed that among my friends, those who study psychology (X1) tend to be less aligned with my own political views (X2). The collider is being friends with me (Y): Psychology students are more likely to become friends with me because, duh, that’s how you find your friends as a student (X1->Y). People who share my political views are more likely to become friends with me (X2->Y). Looking at my friends, they are either psych peeps or socialist anti-fascist freegan feminists.[17]This might sound like I want to imply that the other authors of this blog are fascists, but that wasn’t true last time I checked. Even though those two things are possibly positively correlated in the overall population,[18]Actually, I’m pretty damn sure that the average psych student is more likely to be a socialist anti-fascist freegan feminist than the average person who is not a psychology student. the correlation in my friends sample is negative (X1 and X2 are negatively correlated conditional on Y).
Other examples: I got the impression that bold claims are negatively correlated with methodological rigor in the published psychological literature, but maybe that’s just because both flashy claims and methodological rigor increase chances of publication and we just never get to see the stuff that is both boring and crappy?[19]This might come as less of a surprise to you if you’re a journal editor because you get to see the whole range.
At some point, I got the impression that female (X1) professors were somewhat smarter (X2) than male professors, and based on that, one might conclude that women are smarter than men. But female professors might just be smarter because tenure (Y) is less attainable for women (X1->Y)[20]For whatever reason, you can add mediators such as discrimination and more likely for smart people (X2->Y), so that only very smart women become professors but some mediocre males can also make it. The collider strikes again!
Tenure and scientific eminence are nice examples in general because they are colliders for a fuckload of variables. For example, somebody had suggested that women were singled out as instances of bad science because of their gender. Leaving aside the issue whether women are actually overrepresented among the people who have been shamed for sloppy research,[21]I actually have no clue whether that’s true or not, I just don’t have any data and no intuition on that matter such an overrepresentation would neither tells us that women are unfairly targeted nor that women are more prone to bad research practices.[22]Notice that both accounts would equal a causal effect of gender, as the arrows are pointing away from “gender” and end at “being criticised for bad research”, no matter what happens in between. Of course, the parts in between might be highly informative. Assuming that women (X1) have worse chances to get into the limelight than men, but overstating the implications of your evidence (X2) helps with getting into the limelight; we could find that women in the limelight (conditioning on Y) are more likely to have overstated their evidence because the more tempered women simply didn’t make it. That’s obviously just wild speculation, but in everyday life, people are very willing to speculate about confounding variables, so why not speculate a collider for a change?
Which leads to the last potential collider that I would like you to consider. Let’s assume that the methodological rigor of a paper (X1) makes you more likely to approve of it as a reviewer. Furthermore, let’s assume that you – to some extent – prefer papers that match your own bias (X2).[23]For example, I believe that the metric system is objectively superior to others, so I wouldn’t approve of a paper that champions the measurement of baking ingredients in the unit of horse hooves. If you think I chose this example because it sounds so harmless, you haven’t heard me rant about US-letter format yet. Even if research that favors your point of view is on average just as good as research that tells a different story (X1 and X2 are uncorrelated), your decision to let a paper pass or not (Y) will introduce a negative correlation: The published papers that match your viewpoint will on average be worse.[24]Plomin et al. claimed that the controversy surrounding behavioral genetics led to the extra effort necessary to build a stronger foundation for the field, which is the flipside of this argument.
So peeps, if you really care about a cause, don’t give mediocre studies an easy time just because they please you: At some point, the whole field that supports your cause might lose its credibility because so much bad stuff got published.
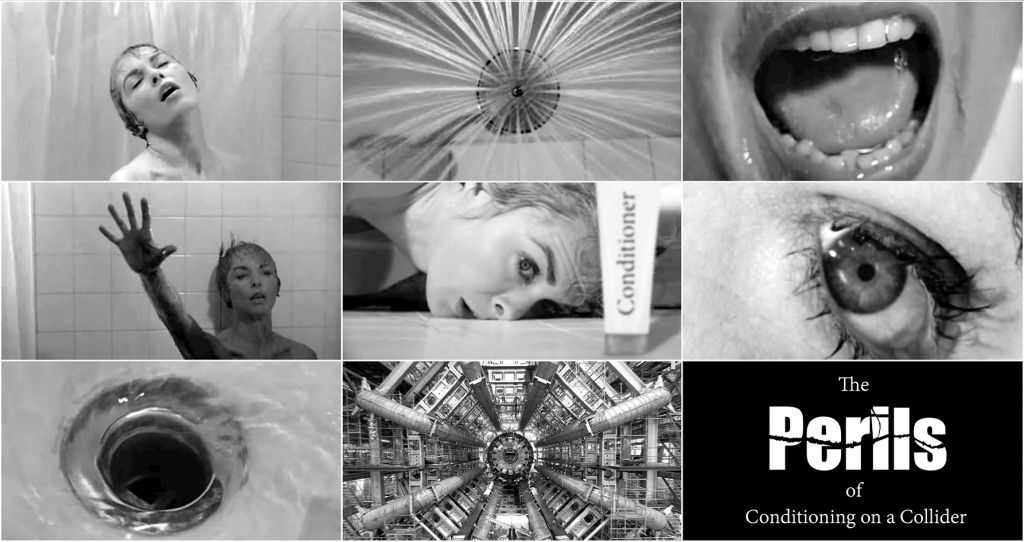
Addendum: Fifty Shades of Colliders
Since publishing this post, I have learned that a more appropriate title would have been “That one weird third variable problem that gets mentioned quite a bit across various contexts but somehow people seem to lack a common vocabulary so here is my blog post anyway also time travel will have had ruined blog titles by the year 2100.”
One of my favorite personality psychologists,[25]Also: One of the few people I think one can call “personality psychologist” without offending them. Not sure though. hides Sanjay Srivastava, blogged about the “selection-distortion effect” before it was cool, back in 2014.
Neuro-developmental psychologist Dorothy Bishop talks about the perils of correlational data in the research of developmental disorders in this awesome blog post and describes the problems of within-groups correlations.
Selection related to phenotypes can bias correlations in genetic studies which has be pointed out by (1) James Lee in Why It Is Hard to Find Genes Associated With Social Science Traits by Chris Chabris et al. and (2) in Collider Scope by Marcus Munafò et al.
Last but not least, Patrick Forscher just started a series of blog post about causality (first and second post are already up), starting from the very scratch. I highly recommend his blog for a more systematic yet entertaining introduction to the topic![26]No CERN jokes though. Those are the100.ci-exclusive!
Update: In the meantime, I have written an article loosely based on this blog post which gives a somewhat more formal introduction to issues of causal inference. Check out the preprint, Thinking Clearly About Correlations and Causation: Graphical Causal Models for Observational Data
Footnotes
↑1 | If you only see footnotes, you have scrolled too far. |
---|---|
↑2 | YMMV and I hope that there are psych programs out there that teach more about causal inference in non-experimental settings. |
↑3 | “Experimental studies are needed to determine whether…” |
↑4 | Added bonus: After reading this, you will finally know how to decide whether or not a covariate is necessary, unnecessary, or even harmful. |
↑5 | I have been informed that only grad students can afford to actually read stuff, which is kind of bad, isn’t it? |
↑6 | There’s no free lunch in causal inference. Inference from your experiment, for example, depends on the assumption that your randomization worked. And then there’s the whole issue that the effects you find in your experiment might have literally nothing to do with the world that happens outside the lab, so don’t think that experiments are an easy way out of this misery. |
↑7 | Just to make sure: I don’t endorse any form of animal cruelty. |
↑8 | If you are already aware of colliders, you will probably want to skip the following stupid jokes and smugness and continue with the last two paragraphs in which I make a point about viewpoint bias in reviewers’ decisions. |
↑9 | As we say in German: “Gönn dir!” |
↑10 | Just to make sure: This is fake data. Fake data should not be taken as evidence for the actual relationship between a set of variables (though some of the more crafty and creative psychologists might disagree). |
↑11 | The collider problem is just the same for continuous measures. |
↑12 | Coincidentally, you will find each of the four combinations represented among the members of The 100% CI at any given point in time, but we randomly reassign these roles every week. |
↑13 | Ha, ha, ha. |
↑14 | Notice that you might be very well able to replicate this association in every college sample you can get. In that sense, the negative correlation “holds” in the population of all college students, but it is a result from selection into the sample (and not causal processes between conscientiousness and intelligence, or even good old fashioned confounding variables) and doesn’t tell you anything about the correlation in the general population. |
↑15 | If you are really good at guessing correlations (it’s a skill you can train!) you might even see that it’s about r = -.200, |
↑16 | or anything that is caused by Y, because the whole collider logic also extends to so-called descendants of a collider |
↑17 | This might sound like I want to imply that the other authors of this blog are fascists, but that wasn’t true last time I checked. |
↑18 | Actually, I’m pretty damn sure that the average psych student is more likely to be a socialist anti-fascist freegan feminist than the average person who is not a psychology student. |
↑19 | This might come as less of a surprise to you if you’re a journal editor because you get to see the whole range. |
↑20 | For whatever reason, you can add mediators such as discrimination |
↑21 | I actually have no clue whether that’s true or not, I just don’t have any data and no intuition on that matter |
↑22 | Notice that both accounts would equal a causal effect of gender, as the arrows are pointing away from “gender” and end at “being criticised for bad research”, no matter what happens in between. Of course, the parts in between might be highly informative. |
↑23 | For example, I believe that the metric system is objectively superior to others, so I wouldn’t approve of a paper that champions the measurement of baking ingredients in the unit of horse hooves. If you think I chose this example because it sounds so harmless, you haven’t heard me rant about US-letter format yet. |
↑24 | Plomin et al. claimed that the controversy surrounding behavioral genetics led to the extra effort necessary to build a stronger foundation for the field, which is the flipside of this argument. |
↑25 | Also: One of the few people I think one can call “personality psychologist” without offending them. Not sure though. hides |
↑26 | No CERN jokes though. Those are the100.ci-exclusive! |
An introductory book specialized in graphical model approach in causal inference is, Causal Inference in Statistics: A Primer, by Judea Pearl, Madelyn Glymour and Nicholas Jewell. Highly recommend it.
Thanks for the great tip! I have to admit I haven’t read that one because I ended up reading Morgan & Winship first and thought it would be pretty redundant (would it, though? maybe I should still read it), but I loved all Pearl papers I’ve read so far. An alternative title for this post was something along the lines of “Pearl before Swine: Invalid causal inference in psychology” 😉
That would be a great title! ?
The Primer book focuses on the graphical model framework and doesn’t cover some estimation methods, such as matching, as well as other specialized models present in Morgan & Winship. It is a theory-inclined introductory book equipped with examples. There are some materials not covered in the Morgan & Winship book, like causal mediation and causal interpretation of SEM, but covered in the Primer book (not in great detail though, IMO). So if you’ve read Morgan & Winship plus many other papers on this topic, it would be redundant to read the Primer book. But it is still a great read if you want to refresh your memory of the graphical model framework.
Thanks for an excellent and readable explanation. I shall recommend this to my students/postdocs.
Your readers might be interested in a related blogpost I did on correlations which in some ways is complementary to this one (though I suspect less easy to follow): http://deevybee.blogspot.co.uk/2012/06/causal-models-of-developmental.html
Thank you for the nice feedback 🙂 And great blog post. I’m planning to write a short addendum because I’ve been directed to multiple related articles and I will include yours as well. It really looks like causality is not covered sufficiently in the average psychology curriculum. We even lack a coherent vocabulary to talk about causality, although other domains (sociology, economics, and Judae Pearl who probably counts as his own scientific domain of causal inference) have put a lot of thought into these things. Yet another reason to keep looking for input from outside of psychology!
I’ve considered writing another post about causal inference in developmental psychology because there are a lot of interesting things to talk about. e.g. should we control for time-varying covariates when plotting age trajectories? I just read in the Observer that “(…) encouragingly, by the time your are 70, if you are still physically fit then on average you are as happy as a 20 year old” and that really made me wonder whether people have any clue what an age effect is supposed to be. I will probably browse your blog for some input 🙂
Ever since we talked about this on Facebook Julia, I keep thinking about this for my studies. Incidentally, we just submitted a paper in which we argue people really should control for all sorts of things, and rather err on the side of controlling too much than too little. Luckily, colliders are unlikely here because one of the two variables of interest is mortality (which rarely causes other psychological behaviors lateron in the same person) ;).
Fantastic blog, well done!
Thanks! Uhm yeah, mortality is unlikely to cause something. Unless…well let’s not talk about the undead!
I don’t think the “just control for everything” approach is necessarily wrong, it’s just that you have to know what the resulting numbers mean and which assumptions render them meaningful. Looking forward to reading your paper, maybe you can convince me that TOTAL CONTROL is the way to go 😉
Generally speaking, I’d would like to see more papers that report associations for different model specifications, from the zero-order correlation to “we just threw in everything we had” so that readers get a fuller picture of the data. Ruben and I wanted to write about sensitivity analyses, so we will likely dedicate another post to these issues.
Yup good idea. In the pairwise markov random fields we use, everything is always thrown in of course (because sim work shows it works really well uncovering the true structure), but you get an idea for collider situations after a while (“aha, a triangle of x1 x2 x3 with strong positive edges x1-x2 and x2-x3 but a strong negative edge x1-x3 — collider alert!”).
Isn’t this related to Simpson’s paradox?
It is! There’s a paper on the “deconstruction” of paradoxes, as most of them can be rephrased in Directed Acyclic Graphs: https://www.ncbi.nlm.nih.gov/pubmed/26164615 (haven’t read it yet, but I agree with the abstract). Pearl also wrote one about Lord’s paradox that helped me understand the difference between comparison of difference scores vs. regression with control for t1 (http://ftp.cs.ucla.edu/pub/stat_ser/r436.pdf). I really appreciate the coherent framework that DAGs offer for all these phenomena, sort of blew my mind when I first learned about it.
Thanks for this background info Julia. I didn’t know about the DAG approach! Fascinating!
Very nice post! I was left wondering whether collider is another way to call suppressor variables (two nice xamples are illustrated here http://ericae.net/ft/tamu/supres.htm), the difference being whether you are interested in X1 vs X2 or X1 vs Y, but maybe it is something different.
According to this discussion it’s something different http://stats.stackexchange.com/questions/33888/x-and-y-are-not-correlated-but-x-is-significant-predictor-of-y-in-multiple-regr
I tried really hard to phrase an answer but I’m a bit unsure myself, so maybe it’s more useful to point you towards some papers that might help:
Simpson’s Paradox, Lord’s Paradox, and Suppression Effects are the same phenomenon – the reversal paradox: https://www.ncbi.nlm.nih.gov/pmc/articles/PMC2254615/
The role of causal reasoning in understanding Simpson’s paradox, Lord’s paradox, and the suppression effect: covariate selection in the analysis of observational studies: https://www.ncbi.nlm.nih.gov/pmc/articles/PMC2266743/
Okay! Now I know how to say it.
As far a I can tell, suppression is used in a purely statistical manner. Say X1 and X2 don’t have a zero-order with Y (or only a very weak one) but if you include them into the model together, you suddenly find an association.
In contrast, a collider is a specific phenomenon when X1 and Y are or are not correlated in some way, but when you include X2, which is both causally influenced by X1 and Y in some manner, the relationship between X1 and Y changes in some way. So the collider only make sense when you are talking about some causal processes, which will necessary happen within a certain model of reality.
Example with content: Let’s assume intelligence (X1) is not correlated with productivity (Y) at an assembly line. Carefulness (X2) is also not correlated with productivity (Y) on its own. However, there is a strong negative correlation between X1 and X2. If you include both into the model, you suddenly might find an association with Y, and that would be suppression.
However, what that “means” from a causal perspective depends on what exactly is happening between the variables. For example, people high in intelligence might actually be potentially much more productive (X1–>Y positive, holding everything else constant). However, they are aware of that, and thus are less careful because they can “afford it”, and this has a negative effect on its own (X1->X2->Y). The positive effect of intelligence on productivity is “negated” by a negative effect of less care. The positive effect of carefulness on productivity is not visible because the careful people in your sample happen to be the less intelligent ones.
Now when you include both in the model, what are you actually doing? If you are interested in the effect of carefulness on productivity, it’s the right thing to do: intelligence is a confounder (X2<-X1->Y; so there is an open “backdoor path” between X2 and Y via X1). Controlling for intelligence, you correctly find that carefulness is actually positively associated with productivity.
But if you are interested in the overall effect of intelligence on productivitiy, it’s the wrong thing to do because productivity is on a causal path from intelligence to productivity (X1->X2->Y), and you would control away part of the effect you are interested in. You would actually be controlling for a mediator of the effect and only estimating what’s left over and then find a positive effect of intelligence. In terms of mediation, you are estimating the direct effect (which would be positive) net of an indirect effect (which is negative). However, if you want the causal effect of intelligence on productivity, you would want to include all pathways from intelligence to productivity, including the one mediated via carefulness.
So suppression describes a statistical phenomenon but talking about confounders, mediators and colliders also assigns certain causal roles to the involved variables.
excellent explanation, thanks a lot!
From your explanation: a collider is a variable that is caused by the predictor and by the outcome; cf. confounder, which causes both predictor and outcome, and mediator, caused by predictor and in turn causes outcome. Colliders and mediators should not be used as control variables, confounders should. Is that correct?
Correct! I just found the figure I sketched for Eiko once: http://www.the100.ci/wp-content/uploads/2017/03/DAG.jpg
I’m not sure about mediators being collidors (I.e. I don’t think so). Julia, could you clarify?
Which part of the comment are you referring to? Maybe I misread. A mediator is not a collider, but it should not be controlled for (unless you want to have the effect net of the mediator).
Hi, thanks, liked this.
p.s. I found your last reply to Daniele was the easiest to follow explanation/example of this phenomena (and suppressor variables) i’ve come across. Given that I’m an academic and have no background in assembly lines it is odd for that example to be the clearest for me (and so maybe lots of other people too), but there you ; )
Eric
Thanks 🙂 I got that assembly line example from Wikipedia. Somehow, I’ve hardly ever encountered an example for statistical suppression that actually assigned meaning to the variables.
Yeh me too. Been trying to wrap my head around what a collider is for the last several days to no avail. And now I reckon I get it. Thanks Julia. 🙂