Content warning: half-assed philosophy of science
Part I: Causal Inference
I am not very keen to join the stats wars, but if I had to join, I would rally under the banner of House Cause. That is the one framework I’d champion in a (randomised controlled) trial-by-combat if necessary: Authors should spell out their analysis goal (their estimands) as clearly as possible. In particular, they shouldn’t be weasley when it comes to causality:[1]like those wimps of House Granger If what you are interested in looks like a causal effect, has the same implications as a causal effect, and quacks like a causal effect, then it probably is a causal effect. Furthermore, authors should explicitly spell out the assumptions under which the data can inform us about the theoretical estimand of interest.
In many cases, when the goal is to infer a causal effect and the data are observational, these assumptions will have to be quite strong. Usually, the more complex the estimand, the worse it gets. A total (i.e., totally vanilla) causal effect from observational data? That is going to involve the assumption that there are no unobserved confounders, which tends to be hard to defend, in psychology at least. An indirect (i.e., mediated) effect from observational data? That will actually be the combination of two causal effects, so now we need to make assumptions about a lack of confounding between multiple pairs of variables. Moderated mediation from observational data, in which we want to figure out how some third fourth variable affects those paths? That’s even worse, and you should feel worse.

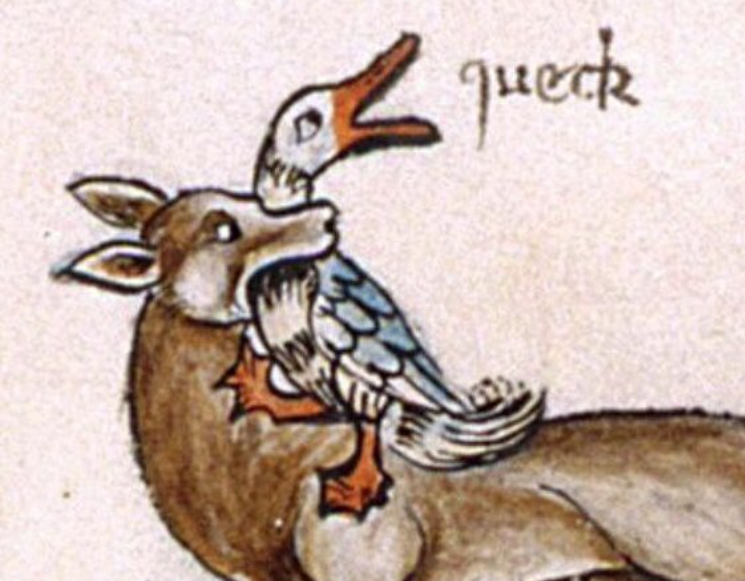
Or maybe you don’t, in which case there is a recent article by us[2]The 75% CI and Paul on the matter. Some time ago, the Journal of Media Psychology linked our article in a tweet in which they stated that they had been desk-rejecting a lot of manuscripts looking at mediation in cross-sectional data.
Quite a few people were unhappy about this tweet because they assumed it implied that all cross-sectional mediation analysis shall be desk-rejected henceforth, no matter the details. The intended message of the tweet was, of course, more nuanced. As it should be! After all, mediation analysis on the basis of cross-sectional, observational data is not impossible per se. It’s just that it is not very convincing in like 90% of cases.
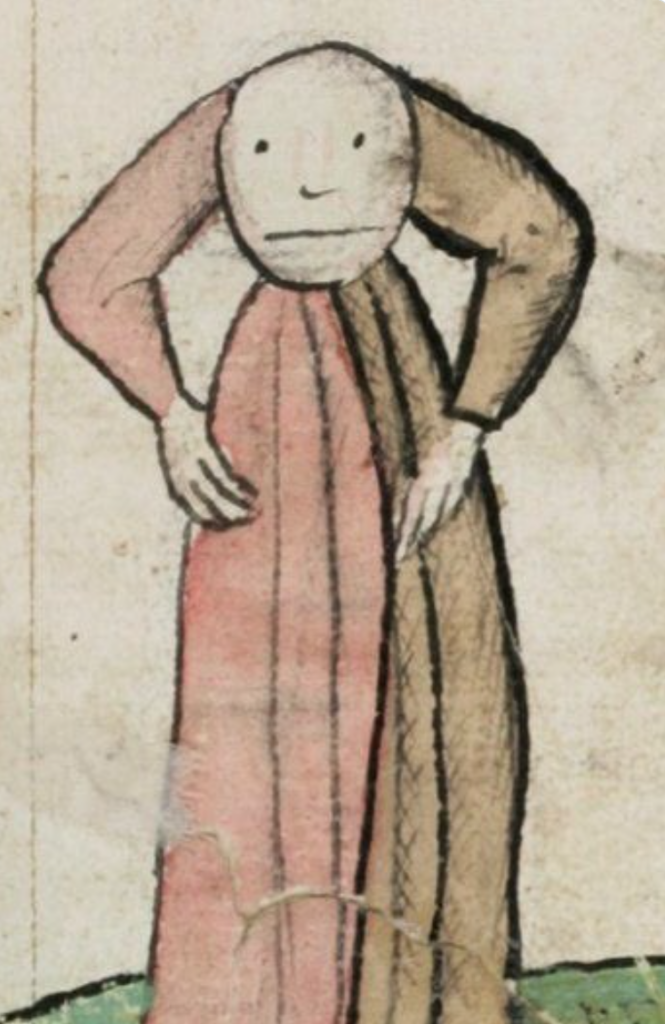
But whenever people start to argue about mediation analysis, there is one particular defense that I find particularly intriguing and worth discussing:
Part II: Hypothesis Testing
Cross-sectional, observational mediation analysis is not about estimating or even inferring causal effects – that is impossible with observational data, by design. However, one may hypothesize a particular mechanism that implies an indirect effect. Now the correlations in the data may be compatible with such an indirect effect, and that should provide some evidence for the hypothesis. After all, my hypothesis predicts something (a correlational pattern), and then that prediction pans out.
This can be leveraged as a defense against anybody who generally criticizes causal inferences on the basis of observational data. Sure, correlation does not imply causation, but causation implies a specific pattern of correlations, and that must count for something. From that perspective, we don’t really need causal inference, do we? We just need a theory that makes predictions, and then we check whether the data align with them.[3]Indeed, I once heard somebody voice confusion about the whole notion of causal inference – what would it be good for? And all those pesky assumptions somehow seem to have disappeared, or at least they appear less central.
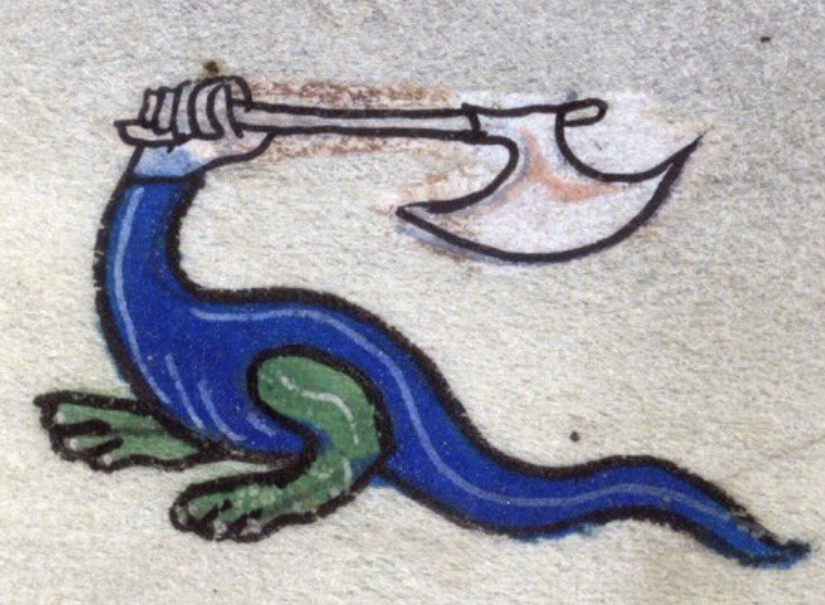
From this angle, one may even think that the more complex the estimands, the better. If we hypothesize that there’s a particular causal effect of X on Y, that may just imply a simple correlation between the two. But if we are interested in a more complex causal chain, that implies a whole pattern of correlations. So our prediction becomes more specific, and if our data confirm these increasingly specific predictions, it just feels like we should become more confident in the underlying hypotheses. But before, we said that more complex estimands require more assumptions and are thus harder to defend. What gives?
Part III: All at Once
[Trying to estimate a causal effect from observational data] and [Testing whether observational data are aligned with some (causal) hypothesis] may seem like different inference games with different rules. What ties the two together is something you may have noticed was missing in the previous part: Testing a hypothesis is actually not so much about trying to confirm it, but about trying to falsify it. Falsification is what makes hypothesis testing powerful in the first place – it’s not very impressive to find support for your hypothesis in a study that had no chance of falsifying it.[4]This is what Deborah Mayo calls “bad evidence, no test” (BENT).
The missing ingredient to make hypothesis testing spicy is the notion of test severity, which tells you the extent to which an empirical observation (e.g., a correlation between X and Y) corroborates a hypothesis (e.g., X has a causal effect on Y). Roughly speaking, an empirical observation provides a severe test of a hypothesis if it is (1) very likely to occur if your hypothesis is true and (2) very unlikely to occur otherwise. Test severity can be formalized as p(observation|hypothesis, background knowledge about the world) divided by p(observation|background knowledge about the world). The higher the probability of the observation given your hypothesis plus any background knowledge about the world, p(observation|hypothesis, background knowledge), the higher the severity (“my theory said so!”). The higher the probability of the observation given just your background knowledge, p(observation|background knowledge), the lower the severity. A finding that makes you go “no shit, Sherlock” (high probability given background knowledge) is unlikely to provide a severe test for some novel and counterintuitive hypothesis about the world.
For example, let’s say your hypothesis is that subjective feelings of happiness improve health. Your empirical observation is that people who say they are happy also report that they are healthy. But everything that you know about the world already tells you that happiness and health should be positively correlated. So the denominator will be large and thus test severity will be low. You’ll need something stronger to support your claim about happiness and health.[5] e.g., a shot of Stroh-Rum
Now that we have the concept of test severity, we can link back to the causal inference framework. If we want to use the observed correlation between happiness and health as an estimate for the causal effect of happiness on health, we have to assume that those two variables are not confounded and that there is no reverse causality with health affecting happiness. Those are, of course, unrealistic assumptions. And these unrealistic assumptions are what render the observed correlation a weak test of the causal effect of interest. Precisely because we know that these assumptions do not hold (for example, because of common causes), we already expect a correlation between the variables. So the denominator, p(observation|background knowledge) will be high and severity will be low.
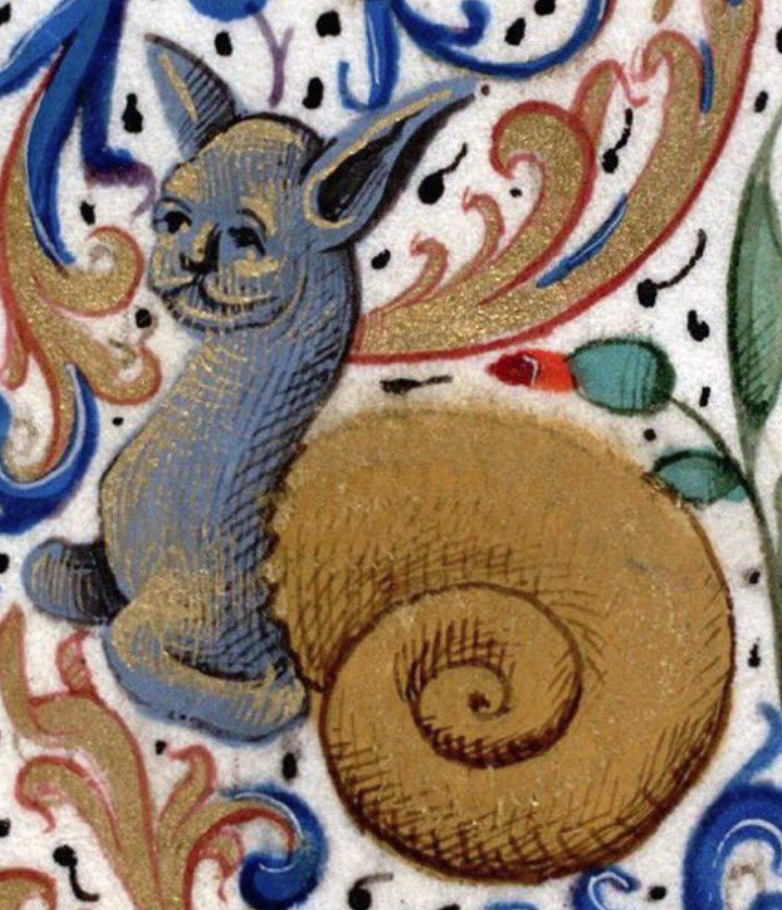
Now, before we talked about mediation, how does this work out here? When people criticize cross-sectional observational mediation analysis, it is often because at least one of the paths is trivially explained without the causal effect of interest. For example, a common pattern involves a psychological mediator and then some vaguely related psychological outcome, both assessed via self-report. Those variables are almost guaranteed to be correlated to some degree, because they may be affected by shared response biases, or by underlying personality traits. So usually, that part of the mediation chain is not subjected to a severe test, and without it, the whole mediation thing turns moot. Of course, often the first path (“independent” variable to mediator) suffers from the same issue, which doesn’t improve the situation. The severity of your mediation test ends up twice as much as nothing at all, which is still nothing at all.
To sum it up: the assumptions necessary to estimate a causal effect from data correspond to the severity of the test that the data provide for the hypothesized effect. If you have to make unrealistic assumptions (that violate your background knowledge), that means that the data provide a weak test of the effect (because said background knowledge already explains the association). If some data seem like a weak test because of an obvious alternative explanation (some background knowledge that “explains away” the observation without the need to invoke the effect of interest), the resulting estimate of the effect will rely on the assumption that the alternative source of association doesn’t exist. What makes or breaks the inference, regardless of whether you frame it as an attempt to estimate a causal effect or as an attempt to test some causal hypothesis, is the same: the other stuff that you know about the world. Curse you, background knowledge!
In the end, with some simplification, saying “those are unrealistic assumptions” is like saying “that’s a super weak test.[6]I have only talked about the case in which the hypothesis is “effect X on Y exists.” That may be the most relevant case for psychology in its current state, but I believe the correspondence holds up more generally for e.g., hypotheses about the absence of effects, directed hypotheses. There are interesting implications for how to think about sensitivity analyses along the lines of “how large would confounding influences need to be to flip the sign of the estimate?” and for experimental studies in which questions are less about the presence of effects and more about the specific mechanisms. I have a truly marvelous discussion of these issues which this footnote is too narrow to contain. Maybe this correspondence seems trivial, but some researchers seem, at the same time, (1) very averse to assumptions (“Unconfoundedness? At this time of year? In this field of research?”) and (2) opportunistically open to weak tests (“Sure it is no conclusive proof, I mean nothing is ever conclusive in science, but the data are compatible with this story”).
Now I don’t believe that weak tests are necessarily worthless, just like I don’t believe that causal estimates that rest on implausible assumptions are necessarily worthless. What seems important to me is that the matter is discussed transparently. And this is precisely why I find the assumptions framing a bit neater: explicitly stating that an analysis rests on the assumption that X and Y don’t share any common causes except for A, B and C makes it very easy for readers to consult their background knowledge and check whether they consider that plausible. So personally, I don’t believe that cross-sectional observational mediation analyses should be desk-rejected by default. But papers that don’t make any effort to be transparent about the underlying assumptions, or that try to sell a weak test as something more severe? Maybe those should be sent back by default.
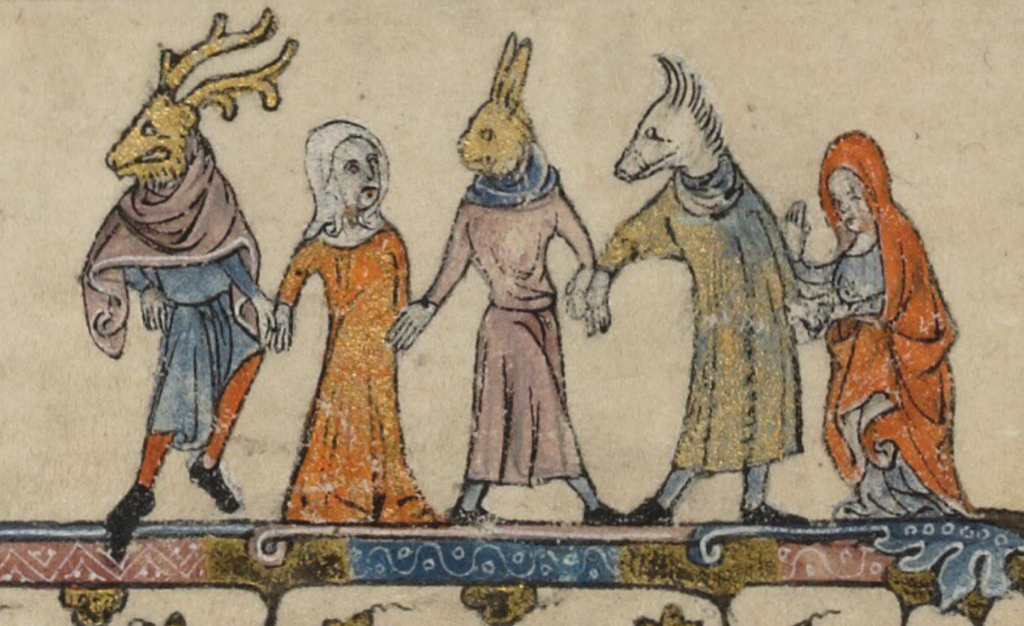
Footnotes
↑1 | like those wimps of House Granger |
---|---|
↑2 | The 75% CI and Paul |
↑3 | Indeed, I once heard somebody voice confusion about the whole notion of causal inference – what would it be good for? |
↑4 | This is what Deborah Mayo calls “bad evidence, no test” (BENT). |
↑5 | e.g., a shot of Stroh-Rum |
↑6 | I have only talked about the case in which the hypothesis is “effect X on Y exists.” That may be the most relevant case for psychology in its current state, but I believe the correspondence holds up more generally for e.g., hypotheses about the absence of effects, directed hypotheses. There are interesting implications for how to think about sensitivity analyses along the lines of “how large would confounding influences need to be to flip the sign of the estimate?” and for experimental studies in which questions are less about the presence of effects and more about the specific mechanisms. I have a truly marvelous discussion of these issues which this footnote is too narrow to contain. |
“The missing ingredient to make hypothesis testing spicy is the notion of test severity, which tells you the extent to which an empirical observation (e.g., a correlation between X and Y) corroborates a hypothesis (e.g., X has a causal effect on Y). Roughly speaking, an empirical observation provides a severe test of a hypothesis if it is (1) very likely to occur if your hypothesis is true and (2) very unlikely to occur otherwise.”
I really liked the way Meehl talked about this topic, in terms of risky tests and money in the bank. Specifically he said that if you perform a risky test—one that has the power to lead to the rejection (or, if we don’t follow Popper too much, modification of a theory)—and your theory passes, the theory gets more money in the bank than when your theory predicts data that really couldn’t look any other way. Theories can get money by 1) predicting things unlikely absent of the theory, i.e. risky successes, or by 2) near-misses (either of which are damn strange coincidences).
In my own work I use traffic jams as example for risky tests: predicting that there will be traffic at some point in NYC is not risky, predicting that there will be a jam at this and this junction at this and that exact time is more risky, and your latter theory would get more money the bank.
Interestingly, theories can get money in the bank even when they are falsified (because they can still have very high versimilitude—again, Popperian dichotomy doesn’t help thinking clearly here imo).
Excellent